Abstract
In this paper, we reported on recent findings from our research on investigating the link between a person’s standard biochemistry profile (based on blood exams), body mass index (BMI), metabolism as health state and Systolic Blood Pressure (SBP). Our current findings expand upon our previous related research, which was based on the use of deep neural networks and other machine learning paradigms in relation to BMI and nutrition [1,2,3,4].
The motivation for this research was to compress tasks and improve outcomes by using more common variables to predict health states and, in a sense, simplify the patient’s journey via minimizing response time between test, result and recommended action. At the same time, another motivation was to find methods to optimize operational issues via applying machine learning methodologies that can be easily transcribed into telemedicine applications. For example, Big Data and artificial intelligence can be used to improve decision making, support interventions [5] and add more pathways in healthcare analytics [6]. Mathematical tools and Big Data, as part of advanced machine learning pipelines and artificial intelligence, will eventually become the basis for analysis in diagnostics and pathology [7].
The process and motivation of this study can be summarized by the following two figures.
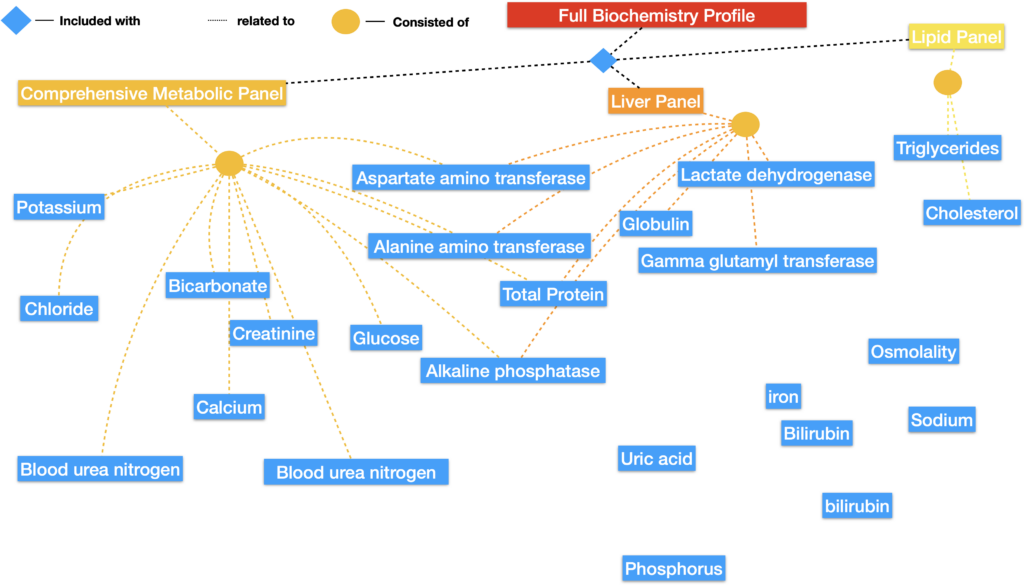
Semantic Grouping of Features for Predictive Modeling in Healthcare(…)
Benchmarks
Metrics | 4 Class Neural Network [5] | 4 Class SVC | 3 Class Neural Network [6] | 3 Class SVC | Cascaded SVM | Extreme LM [12] |
Accuracy (%) | 56 | 55 | 58 | 62 | 85 | 90.54 |
Size of sample | 75,000 | 15,000 | 33,000 | 15,000 | 10,000 | 500 |
Balanced sample | no | no | yes | yes | yes | no |
All Classes? | yes | yes | no | no | yes | no (Overweight class) |
Features | Full Biochemistry Profile | Full Biochemistry Profile | Full Biochemistry Profile | Full Biochemistry Profile | Without defining factors of MetS (15 features) | Blood Indexes (39 features) + age |
Authors
Dimitrios P. Panagoulias, Dionisios N. Sotiropoulos and George A. Tsihrintzis
References
- Thomas, D.; Ravi, K. National health and nutrition examination survey: Sample design. Future Healthc. J.2019, 6, 94. [Google Scholar]
- Strimbu, K.; Tavel, J.A. What are biomarkers? Curr. Opin. HIV AIDS 2010, 5, 463. [Google Scholar] [CrossRef] [PubMed]
- Panagoulias, D.P.; Sotiropoulos, D.N.; Tsihrintzis, G.A. Towards Personalized Nutrition Applications with Nutritional Biomarkers and Machine Learning. In Advances in Assistive Technologies: Selected Papers in Honour of Professor Nikolaos G. Bourbakis—Vol. 3; Tsihrintzis, G.A., Virvou, M., Esposito, A., Jain, L.C., Eds.; Springer: Cham, Switzerland, 2022; Volume 28, pp. 73–122. [Google Scholar]
- Panagoulias, D.P.; Sotiropoulos, D.N.; Tsihrintzis, G.A. Nutritional Biomarkers and Machine Learning for Personalized Nutrition Applications and Health Optimization. Intell. Decis. Technol. 2021, 15, 645–653. [Google Scholar] [CrossRef]
- Panagoulias, D.P.; Sotiropoulos, D.N.; Tsihrintzis, G.A. Nutritional Biomarkers and Machine Learning for Personalized Nutrition Applications and Health Optimization. In Proceedings of the Twelfth IEEE International Conference on Information, Intelligence, Systems and Applications, Chania, Greece, 12–14 July 2021; pp. 731–733. [Google Scholar]
- Panagoulias, D.P.; Sotiropoulos, D.N.; Tsihrintzis, G.A. Biomarker-based Deep Learning for Personalized Nutrition. In Proceedings of the 33rd IEEE International Conference on Tools with Artificial Intelligence (ICTAI), Virtually, 1–3 November 2021; pp. 73–122. [Google Scholar]
- Massaro, A.; Ricci, G.; Selicato, S.; Raminelli, S.; Galiano, A. Decisional Support System with Artificial Intelligence oriented on Health Prediction using a Wearable Device and Big Data. In Proceedings of the 2020 IEEE International Workshop on Metrology for Industry 4.0 IoT, Roma, Italy, 3–5 June 2020; pp. 718–723. [Google Scholar]