Abstract
In this paper, we present a novel Artificial Intelligence (AI) -empowered system that enhances large language models and other machine learning tools with rules to provide primary care diagnostic advice to patients. Specifically, we introduce a novel methodology, represented through a process diagram, which allows the definition of generative AI processes and functions with a focus on the rule-augmented approach. Our methodology separates various components of the generative AI process as blocks that can be used to generate an implementation data flow diagram. Building upon this framework, we utilize the concept of a dialogue process as a theoretical foundation. This is specifically applied to the interactions between a user and an AI-empowered software program, which is called “Med|Primary AI assistant” (Alpha Version), and provides symptom analysis and medical advice in the form of suggested diagnostics. By leveraging current advancements in natural language processing, a novel approach is proposed to define a blueprint of domain-specific knowledge and a context for instantiated advice generation. Our approach not only encompasses the interaction domain, but it also delves into specific content that is relevant to the user, offering a tailored and effective AI–user interaction experience within a medical context. Lastly, using an evaluation process based on rules, defined by context and dialogue theory, we outline an algorithmic approach to measure content and responses.
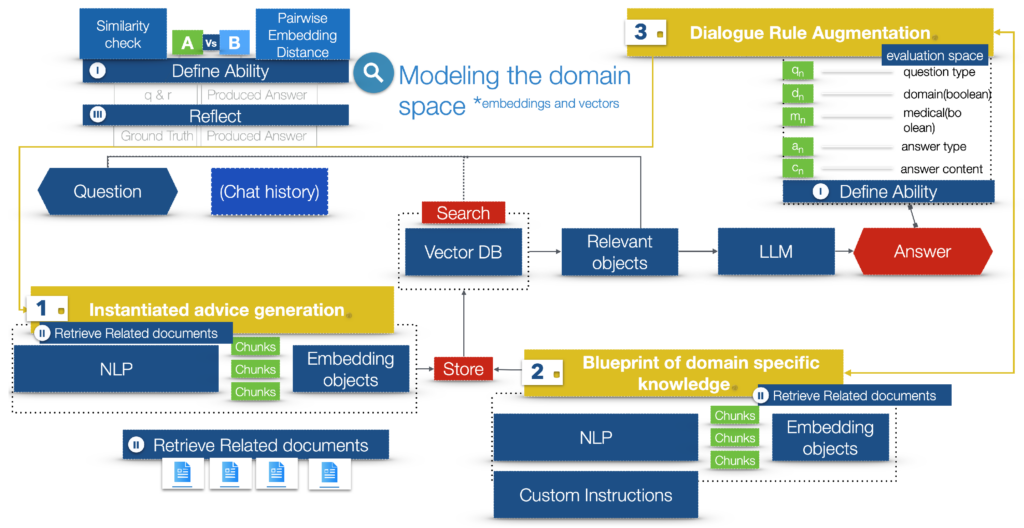
Domain settings, flow chart
Dialogue Augmentation – Evaluation process based on rules
Each component that defines the answer is represented by the appropriate letter. In~a range of (n), a final score would finalize a decision as per the quality of the answer, which in essence represents the ability (A) of the LLM to comprehend the answer and also the effectiveness of the domain setup
Require: Question (q), Domain (d), Medical (m)
Ensure: Answer (a), Grade of Answer Content (c)
1: Begin
2: if (q == “Informational” OR q == “Open-Ended”) AND (d == “Yes”) AND (m == “Yes”)
then
3: a ← “Direct or Elaborated”
4: else if (q == “Any”) AND (d == “No”) then
5: if (m == “No”) then
6: a ← “(deferred) Dialogue Reset”
7: else if (m == “Yes”) then
8: a ← “(deferred) Refer To Specialist”
9: end if
10: end if
11: c ← AnswerContent
12: r ← RetrieveDocuments
13: grade(Ab) ← Define Ability(c)
14: grade(Re) ← Reflect(c)
15: return a, grade
16: End
17: function DEFINEABILITY(AnswerContent)
18: // A grading logic for Answer content(1)
19: // Return grade(Ab)
20: end function
21: function REFLECT(AnswerContent,documents)
22: // A reflection(2) logic for answer content and document retrieval(3)
23: // Return grade(Re)
24: end function
Authors
Dimitrios P. Panagoulias, Maria Virvou and George A. Tsihrintzis