Abstract
The developed approach in this research, centers around personalisation derived from targeted clustering techniques based on the principles of the Technology Acceptance Model – TAM. By employing targeted clustering, we create tailored explanations for users based on their unique characteristics and requirements. To validate the effectiveness of our methodology, we conducted a case study using AI-empowered medical applications. The objectives of this study were threefold:
Highlights
1.Assess and quantify the perspectives of doctors regarding the relevant parameters, such as AI literacy and the level of abstraction in explanations.
2.Generate layers of personalised explainability based on individual user abilities and needs in terms of trustworthiness, ensuring that the explanations are tailored to the users’ specific requirements.
3.Provide a transparent environment that fosters understanding and validation of the AI-empowered medical applications’ decisions, increasing overall trust in the system.
For the purpose of XAI personalisation we proposed, outlined and implemented a novel framework named PINXEL. The acronym stands for Personalised INterface for eXplainability basEd on Levels. The levels we have considered are those of AI explainabilty and abstraction.
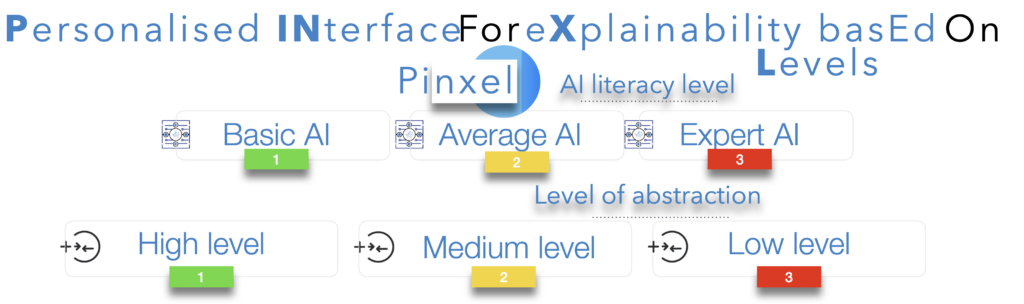
Authors
Dimitrios P. Panagoulias, Maria Virvou, George A. Tsihrintzis